SciBeh 2020 Virtual Workshop on "Building an online information environment for policy relevant science"
9-10 November 2020
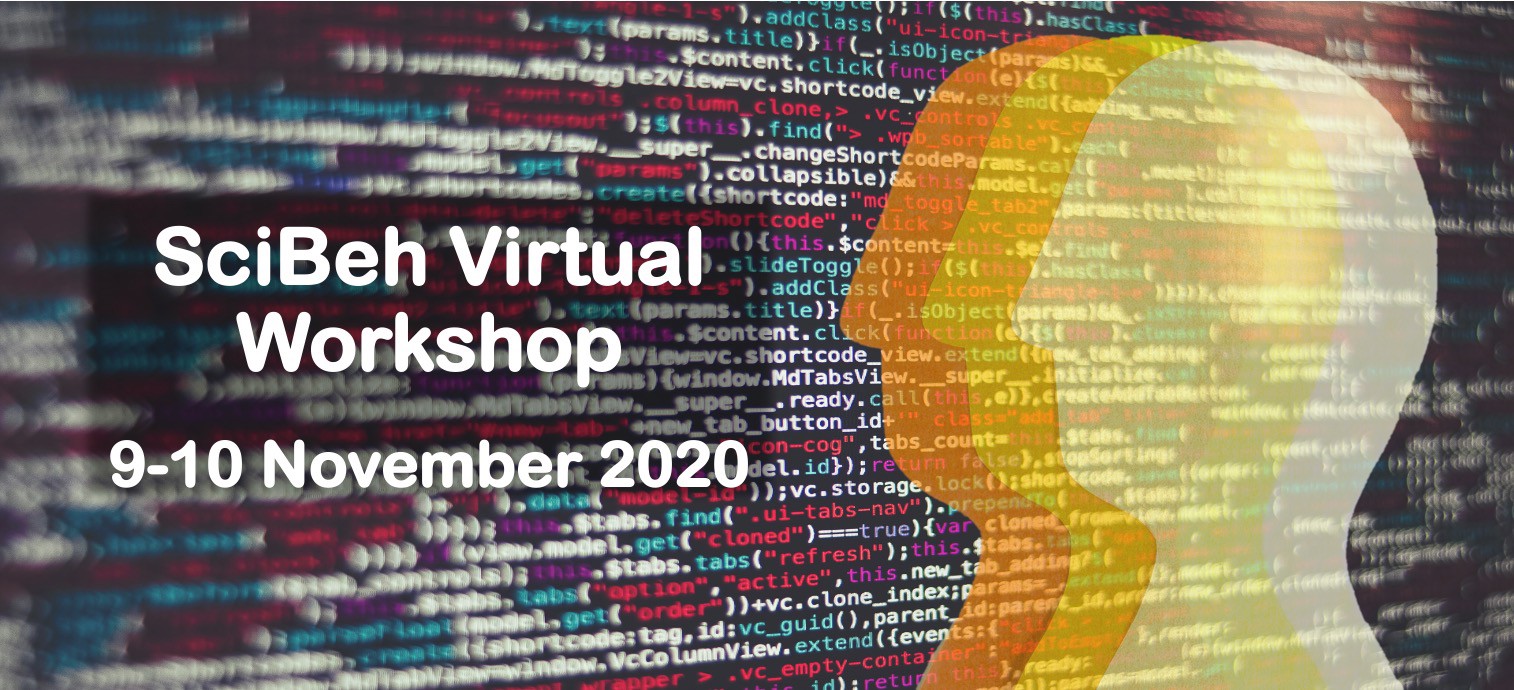
Jump to the following sections:
- Goal
- Organization team
- Workshop outputs
-
Summary Report
- Theme 1: Transparency and openness in research production, dissemination, and curation
- Theme 2: A balance between speed and quality in producing research
- Theme 3: Synthesis of evidence over individual studies
- Theme 4: Communicating research to the public
- Theme 5: Improving the process of critique and review
- Theme 6: Better tools for curating research digitally
- References and resources
Goal
The information environment we need would ensure information that is
- Rapid: facilitating new research, evidence aggregation, and critique in real-time
- Relevant: managing information flood while delivering information in contents and formats that match the needs of diverse users, from scientists to policy makers
- Reliable: generating and promoting high quality content
The workshop brought together an interdisciplinary group of experts and practitioners to help conceptualize, plan and build the tools for such an environment.
Organization team
- Key organizer: Dawn Holford
- Co-organizers: Ulrike Hahn, Stephan Lewandowsky, Stefan Herzog, & Marlene Wulf
- Organisational support: Marta Radosevic, Gaurav Saxena, Erik Stuchly, & Siyan Ye
Outputs
- Sessions Monday
- Sessions Tuesday
- Hackathons
-
#1: Combatting COVID-19 misinformation with lessons from climate change denial
-
#2: Optimising research dissemination and curation
-
#3: ReSearch Engine: Search Engine for SciBeh’s knowledge base & beyond
Try out the prototype of the scibeh search engine. For feedback, please contact us at [email protected].
-
Session 1.1: Open Science and Crisis Knowledge Management
How can we adapt tools, policies, and strategies for open science to provide what is needed for policy response to COVID-19?
- Session overview
- Session chair: Dawn Holford, University of Essex
- Session panelists
- Session summary
- Join the post-workshop discussion
Session 1.2: Interfacing with Policy
How can the wider science community be policy-relevant?
- Session overview
- Session chair: Stephan Lewandowsky, University of Bristol
- Session panelists
- Session summary
- Join the post-workshop discussion
Session 2.0 The Role of Social & Behavioural Science in Pandemic Response
20min session with Martha Scherzer from the World Health Organization: bringing social and behavioural science into COVID-19 and other emergency/outbreak responses (followed by a Q&A-style discussion led by Ulrike Hahn).
Martha Scherzer (World Health Organization) is a Senior RCCE Consultant at the World Health Organization, who has worked on tools for behavioural insights on COVID-19 and managing governmental responses.
Session 2.1: Managing Online Research Discourse
In this session, we address the issue of building sustainable, transparent, and constructive online discourse among researchers as well as between researchers and the wider public.
- Session overview
- Session Chair: Ulrike Hahn, Birkbeck College
- Session panelists
- Session summary
- Join the post-workshop discussion
Session 2.2: Tools for Online Research Curation
We look at what has been done in the past year to aggregate and quality-check new information using machine learning and NLP techniques, and ask what is the next step in delivering robust knowledge to those who need it.
- Session overview
- Session chair: Ulrike Hahn, Birkbeck College
- Session panelists
- Haoyan Huo, UC Berkeley/ CovidScholar
- Hildy Fong Baker, MIT Press/Rapid Reviews: Covid-19
- Jaron Porciello, Cornell University
- Mark Levene, Birkbeck College
- Martyn Harris, Birkbeck College
- Michael Perk, collabovid.org
- Nick Lindsay, MIT Press/Rapid Reviews: Covid-19
- Stefan Herzog, Max Planck Institute for Human Development
- Session summary
- Join the post-workshop discussion
Summary Report
SciBeh’s workshop brought together an interdisciplinary group of experts and practitioners to help conceptualise, plan and build the tools for an information environment for policy-relevant science.
Several themes emerged throughout the talks, panels, discussions, and hackathons. These address how to design and manage this information environment, and the challenges of doing so. In brief, here are six areas to address in the online information environment:
- Transparency and openness in research production, dissemination, and curation
- A balance between speed and quality in producing research
- Synthesis of evidence over individual studies
- Communicating research to the public
- Improving the process of critique and review
- Better tools for curating research digitally
This document summarises the discussions from the workshop under each of these themes (plus references and resources). Contributions to the themes came from multiple sessions.
Theme 1: Transparency and openness in research production, dissemination, and curation
Transparency plays an important role in the research process. It allows research output to be independently scrutinised, not just by other academics, but also the wider public. From a moral standpoint, public money is typically involved in research, and therefore the public should be able to access the output of what they have funded.
Open access to research goes beyond making the final output available. That output needs to be accessible, meaning that it can be understood by non-specialist stakeholders as well. This is particularly important when it comes to understanding the quality of rapid new evidence. Sharing preprints can help the public understand the progressive nature of science, but we need to communicate this process in a clear and consistent manner. We could, for instance, distinguish what it means to talk about “evidence” vs. declaring one’s stance on a matter. This may help avoid backlash on the open sharing of scientific evidence that may clash with a researcher’s personal stance on an issue.
The principles of open science and transparency also include increasing accessibility to doing research. Increasing the diversity of researchers, together with open sharing of research at different stages, would improve the quality of research discourse and diversity of innovations. Further, public trust and research is boosted by having diverse individuals that people can identify with, countering the image of academics in an ivory tower.
Openness about research processes can also help to improve public trust in evidence. This means transparency at earlier stages, such as designing research, registration of experiments, and idea sharing. It allows the public to see into the way scientific evidence aggregation works, including the uncertainties and biases inherent in the process. Especially when machine learning is used to curate evidence for policy, it is important to convey that these are based on human decisions about what metrics are used to search, filter, and rank studies. For example, are we prioritising studies for inclusion based on their citation count and what are the drawbacks here (such as reducing the heterogeneity of evidence returned)? Who gets to determine what an algorithm filters out as “noise” and are we evaluating if this reduces information pollution vs. excludes certain important voices? While machine learning becomes more popular in trying to automate search processes for evidence, it is important to still incorporate machines and humans working together. Machine learning can facilitate processes, but ultimately human input must support the process, assess and feedback on material returned.
How transparent to make a process is still an open question: we may ask whether it is worth trying to explain, especially if it then means people discount the evidence when they know it is not “perfect”. However, people do understand the trade-offs that need to be made in decision-making. For instance, in balancing speed and quality. With machine-learning processes, we may need to explain that algorithms that return answers to a question may be more precise, but also less transparent in how they do so. In cases where such evidence is used in policy decisions, openness about how it is produced can combat the idea that research is produced for political parties.
There are many challenges involved in issues of transparency and open science, but ultimately, greater transparency and accessibility to how scientific evidence is produced facilitates trust and better scientific discourse. Global organisations relying on evidence need the scientific community to propagate these practices. The question should not be whether or what we make transparent, but how we do so, and better explain each of the processes we are sharing to non-specialists.
Theme 2: A balance between speed and quality in producing research
For research to be used in policy decisions and crisis or emergency response, it needs to be rapidly produced and communicated in order to meet the short working time frame in, for example, a policy cycle (that tends to operate in days or weeks). This year, researchers demonstrated the ability to produce output in short timespans: COVID-19 research articles were fast-tracked for publication, submissions proliferated across preprint servers to openly share research results as soon they were available, rather than wait for journal acceptance. However, some of this published evidence were shown to have errors: both in preprints and published journal articles, which had to be retracted after they had been the subject of public scrutiny.
High profile retractions are damaging to science because they devalue work and harm trust in scientific information. Typically, the peer-review process provides critique that helps to maintain scientific rigour in published work in journals (though this does not always function well, since there is no standardised form of reviewing). Preprints, which are shared before a formal review process, are a good way to share new research in a timely fashion—often while they are being submitted to journals—but in the current crisis, many have been picked up by news media and used as scientific evidence to support policy decisions. This highlights a need for some scrutiny of preprints. This needs to be conducted rapidly to match the pace of research production that is necessary to meet the needs of policy challenges.
However, the process of review is unable to keep up with the speed of production. ASAPbio estimates that only 5% of preprints published get spontaneously reviewed by peers. Initiatives like Rapid Reviews Covid-19 have tried to prioritise preprints that need review using machine learning to identify them by topic, and match them to reviewers. Yet this process still only captures a tiny fraction of emergent preprints. Spontaneous review of preprints also do not guarantee that the authors engage with them, nor that other stakeholders read the critique along with the publication.
It is worth noting that while academics cite lack of time as a main reason for not reviewing more, the time needed to review a manuscript (typically measured in hours) is less that the time needed to produce a new piece of research (days, if not weeks or months). The issue may thus be less that of resource availability, but of incentives. Despite the critical contribution of peer review to the scientific process, it is not incentivised in the publication structure, nor by most employers (i.e., universities). This points to an outdated journal system where the metrics and incentives for publishing are no longer aligned with the indicators of good quality scientific evidence. Despite the profitability of the journal industry, the contributors to the system (authors and reviewers) are entirely unpaid (or in the case of authors, may even pay to allow others access to their work).
Researchers need to drive change to the entrenched way of working. However, the willingness to adopt better practices in reviewing and publishing can mean career harm for researchers who go it alone. For instance, prioritising time for reviewing work over producing it may mean hurting one’s chances at securing future employment, permanent academic positions, or promotion. This is analogous to movements for better working conditions, where a critical mass of workers needs to agree at the same time to take actions to better their workplace.
Another area that could help balance the need for speed and quality in producing research is to consider the timing of critique. Conflict is more likely to result over study imperfections after research is conducted than before. Critique about study designs is also highly useful early in the research process, where changes can still be made, than after work is already published. Models such as Registered Reports aim to provide critique at this earlier stage. However, these are still subject to the same reviewing constraints of time and incentives. Pre-registration is another way to apply due process and transparency while research is conducted in a timely fashion. By specifying the research design and analytical plans—and committing to making these publicly available along with the published results—anyone can easily check whether these are followed. Furthermore, pre-registration does not need to conflict with the needs of policy-making. There are examples of pre-registration processes for policy-relevant research.
Trade-offs are necessary to generate new evidence quickly. However, we should question the premise that this means lower scrutiny or less critical review, and examine the flawed incentives that prevent a good balance of research production and peer review.
Theme 3: Synthesis of evidence over individual studies
Scientific evidence is a process of accumulation: researchers consolidate what has been done, and build upon it. Rather than any one individual study, it is the overall, larger body of work that allows us to assess evidence for or against a theory, model, or intervention. Policy-making needs this synthesis of all the available research, which raises the question of how it can be curated in a useful way. A further issue, both for this crisis and others, is that evidence must be drawn from different disciplines in order to develop a holistic response.
Conducting this synthesis is a challenge because it involves searching for relevant information that is appropriately diverse and interpreting the evidence (often coming from different disciplines with each their own terminologies). It is thus more helpful for policy-makers to have access to experts who can synthesise and advise, rather than advocate or supply their own research as evidence. Because of the diversity of evidence sources, there also needs to be diversity in experts consulted. With the short time frames involved, gaining access to evidence (in terms of sources and expert interpretation) tends to involve reaching out to personal connections.
For an information environment to support policy-making, it would need to consider this type of rapid evidence synthesis and how to present it in a less technical format.
Constructing specialised data science tools can be one less time-consuming way to conduct such synthesis and identify diverse expert groups to contact. This is especially the case when the topic area involves work done in highly specialised fields that might not show up in typical web searches because they are less well cited.
Co-production of research can also be a way to design more policy-specific research that collects evidence to support a policy, or evaluate its outcomes with scientific rigour. This can build trust between scientists and policy-makers over a longer period of collaboration, facilitating communications between the two groups. However, there are some concerns here about the independence of research: it is important for academics to retain their research integrity, and not be co-opted into supporting conclusions that are desired. The roles in policy-making can be misunderstood by the public, who do not always make the distinction between civil servants and politicians—public trust in evidence could thus be undermined if scientists are perceived to be ‘working for the government’ by collaborating with policy-makers.
It is also important to note the wider context of research as well. While it is important to build bodies of evidence to synthesise in areas of interest for policy-making, a focus on doing policy-based research would leave a gap in the scientific landscape if other areas are less valued or supported. It would also reduce the diversity of evidence available on the wider topic area. This is still critical to form the overall base of knowledge on which to advance science.
Theme 4: Communicating research to the public
There is increasingly a call for universities and scientists to perform a civic responsibility by engaging in dialogue with public stakeholders of their research activities. Transparency and openness play critical roles in increasing the accessibility of research to wider audiences. Online channels of communication have widened public access to research (e.g., as preprints) and also scientific discourse (e.g., on Twitter).
Sharing research as preprints, which could be thought of as research in an intermediate stage, could help the public understand the evolution of scientific knowledge. However, preprints and/or their findings are occasionally amplified on social media without the full context. This needs to be monitored, especially if news media subsequently pick up ths story without sufficient checks. There may need to be better tools to minimise research being amplified out of context, for example labels that highlight research context and domain specifity so that it does not get wrongly applied (e.g., evidence of treatment for mice getting portrayed as evidence for humans).
Discussing research on social media opens the door to conversations with the public about research, but also critique—often from other scientists who may disagree publicly with the findings or their interpretation. Should these debates be public? There is some evidence that the public does think disagreement among scientists is good and brings us closer to uncovering the true state of things. Like sharing preprints, open scientific debate offers a window into the nuances and uncertainties in science. It is, however, an unresolved question as to whether the public has the capacity and/or motivation to appreciate these nuances and uncertainties without dismissing the scientific findings as impractical or irrelevant. The nature of social media platforms like Twitter also means it is hard to identify who the audience is, and tailor communication to address them. One would not use the same style to explain research to the public as one would to debate with a fellow scientist, but these are both potential audiences for a single social media statement.
It is also worth remembering that ultimately, multiple channels are needed to communicate to different audiences and communities who may be affected by research. Even with social media, there is not a single platform that works for all users. When speaking to communities, involving members of the public that has contributed to these (e.g., from being part of a study) could be a way to build public trust in the evidence shared.
Theme 5: Improving the process of critique and review
For evidence to be useful to policy, it needs to be rigorously produced. Scientific discourse, especially in the form of critique and review, plays a critical role in scientific rigour. As such discourse moves more into online spaces, we increasingly observe heightened conflict from scientific disagreement. Some of this may be a function of the medium of communication: exchanges on social media are asynchronous, lacking the collaborative nature of face-to-face interactions or its built-in tools that help maintain politeness and mutual understanding. Without this conversational ‘glue’ that signals disagreement in non-aggressive ways, disagreements more easily escalete into conflict. There is less awareness of these elements of miscommunication because they tend to be missed in big data analyses of online communications, simply because they are not compared to data from face-to-face interactions.
The asynchronicity of communication means communicators must be more explicit to convey their points, which can be perceived as being impolite, uncaring, or aggressive. Because there are no automatic cues from a conversation partner or audience, exchanges can be more like a series of monologues than a dialogue. This is not an entirely new phenomenon, nor is it restricted to social media discourse. The most traditional form of scientific exchange, journal publications, are also highly asynchronous, and review processes can also spark conflict between scientists (though less openly). Journal publications are even more of a monologue, since once articles are published, critique of them tend not to be published by journals, effectively stopping conversation about the work—unless it is tackled elsewhere, often now on social media.
Online spaces outside of journals now provide these critical functions for critiquing work. These have become more important given the emergence of preprints to make work available that has not yet been critiqued. Online communities are quicker to mobilise in providing critique, e.g., discussing it on Twitter. However, authors often do not engage with the critique, and may dismiss it as less ‘valid’ than that offered by peer review.
Online platforms are also more fleeting in nature than journal publication, where it is accepted that the existing body of work will be appropriately cited (though this can be disrupted and certain work dropped off over time). Scientific discussions on social media do not have the same accumulation, and thus are more likely to be lost.
Some publishing models have tried to adapt through ‘overlay journals’. These identify relevant preprints using machine-learning-driven search and publicly review them. Authors are then invited to publish with the journal based on the outcome of those reviews.
From the perspective of scientific authors, engaging with critique of work in an open online forum can be a minefield. The process of knowledge accumulation involves failure and being wrong, yet academic culture does not sufficiently accommodate the sharing of ideas that could yet be proven wrong (but nonetheless contribute to science). There is also a worry among researchers that sharing ideas too early may result in someone else taking the idea and running with it. Early career researchers and those from minority backgrounds also tend to be penalised more heavily for sharing early-stage ideas. This means that senior scientists may need to take the lead in setting the norms and expectations for an online environment. For instance, being comfortable admitting to being wrong and sharing collective experiences of failed research.
The way we communicate online can also be adapted to adapt cues from face-to-face discussions. For instance, we can moderate our written exchanges by introducing ambiguities and hedges into our writing (e.g., using ‘I think’, ‘maybe’ more often). Social media platforms also offer a chance for increased interactions with users, which can help build trust over time and enable us to interpret the intent behind others’ critique. Finally, we need a culture where critic is appreciated, for instance through formal peer acknowledgement of feedback.
Theme 6: Better tools for curating research digitally
Curating research is useful for better evidence synthesis, but how do we adjust our tools to cope with the rapidly increasing corpus of knowledge? To retrieve relevant knowledge, one needs to be able to segregate noise from signal when running a search.
As an example, if a policy-maker needed to know how masks were beneficial to managing the COVID-19 crisis, papers that study the use of masks in other infectious disease contexts might be relevant, but in a typical search engine, these papers would not necessarily turn up in a search for masks + COVID-19, or even generically with ‘infectious diseases’. Yet searching masks on its own could return plenty of generic results about masks in contexts that are not relevant. The policy-maker might also wish to filter results based on quality of evidence (e.g., on the basis of peer review), but this is also not possible with typical search engines.
Data science and machine learning can be turned towards addressing these current limitations, as several projects (e.g., CovidScholar, Collabovid) are trying to do. Machine learning systems would be trained on human annotations of a known corpus, so that the AI (artificial intelligence) learns the necessary associations to decide what is related to relevant information. In the example above, a specific search tool would need to account for links between COVID-19 and other infectious diseases so that the machine recognises these diseases as relevant to the context of a search for masks and COVID-19. Initial annotation processes are therefore important to cement these associations. However, this also means that if there are biases in the way training data is annotated, or if the available data is historically biased towards one type of research, these biases will become embedded in the algorithms. We thus need to be careful while building and training algorithms. For instance, if we train machines to omit publications by a certain metric (e.g., using ‘citation count’ as a proxy for quality), we have to be very careful that that metric actually correlates with quality rather than popularity of the topic. It should also not leave out other less traditional sources that may be nonetheless important (e.g., reports, preprints). Projects to apply the right tags and labels to different types of work (e.g., SciBeh’s eclectic knowledge base) could enhance their readability by machines and add to the pool of relevant resources.
A further challenge to addressing is that items in a database may be incorrectly filtered out if they contain misspellings, or incorrectly included if they contain keywords that are used in a different context which gives them a different meaning. Words may also change in meaning (or acquire additional ones) over time, so this poses issues when querying databases that go further back in time. There may also be discrepancies between the languages used within two different certain disciplines, as well as the language used by people conducting the search. Tools thus need to be flexible enough to account for these scenarios.
Finally, it is important to support the automated retrieval of information with user-friendly interfaces for querying. Ultimately, one relies on a search engine to return meaningful answers to a question.
These issues reveal the scope of the challenge in tuning data science tools to curate research for better policy relevance. They also highlight the need for human input in the process. Machines are excellent facilitators of search and retrieval, but human judgement and assessment is still needed to evaluate the outputs and feedback on the processes.
References and resources
Publications
- Research integrity: Don’t let transparency damage science
- Combining expert judgment: On the performance of trimmed mean vote aggregation procedures in the presence of strategic voting
- PRINCIPIA: a Decentralized Peer-Review Ecosystem
- Stop explaining black box machine learning models for high stakes decisions and use interpretable models instead
- Understanding and navigating the landscape of evidence-based policy
- Using Evidence: What Works?
- The dos and don’ts of influencing policy: a systematic review of advice to academics
- How can researchers engage with policy?
- COVID 19: Known Unknowns (Facing up to scientific uncertainty during a pandemic)
- Sustainable solutions to end hunger
- Latour, B. (1990). Drawing things together. Representation in scientific practice. MIT Press, Cambrige, MA.
Platforms/Websites
- BETA
- ASAPbio
- Free Our Knowledge
- UPEN
- Gov.uk Areas of Research Interest
- UK Parliament Areas of Research Interest
- CAPE
- Open Innovation Team
- Ceres2030
Tools
- SciBeh Knowledge Base
- Database created by the International Network for Government Science Advice on COVID-19 Evidence-to-Policy
- COVID-19 Social Science Research Tracker
- CovidScholar
- Rapid Reviews Covid 19
- Collabovid